Many companies are opting to create their own large language learning models (LLMs). It provides the ability for customisation and personalisation, control over improvements, and the means to right-size your models in response to your specific requirements, which can lower costs.
But how do custom-built LLMs stack up compared to the big enterprise heavyweights? While established companies like Mistral AI, Silo AI, and Stability AI are creating European alternatives to existing LLMs, one small startup has developed a model that outpaces its predecessors in AI forecasting.
AssetFloow is a Lisbon company with a team of eight, that is developing sensorless behavioural AI software for retailers and FMCG (fast-moving consumer goods). Its forecasting AI provides accurate metrics on shopper journeys and behaviour within stores, without the need for cameras or other sensors, citing a 99% per cent. accuracy rate.
This helps retailers drive sales growth, from customer intelligence to merchandising, eliminate waste and make informed data-driven decisions that enhance operational efficiency, all while prioritising customer privacy.
The company has developed a new AI architecture, Dynamic Flow Network (DFN). Leveraging the Monash Repository, DFN has demonstrated more than 20 per cent improvement in accuracy compared to its predecessors, all while utilising 50 per cent less data for training.
It surpasses established models like Google's WaveNet (2016), Yandex's CatBoost (2017), Meta's Prophet (2017), and Amazon's DeepAR (2018).
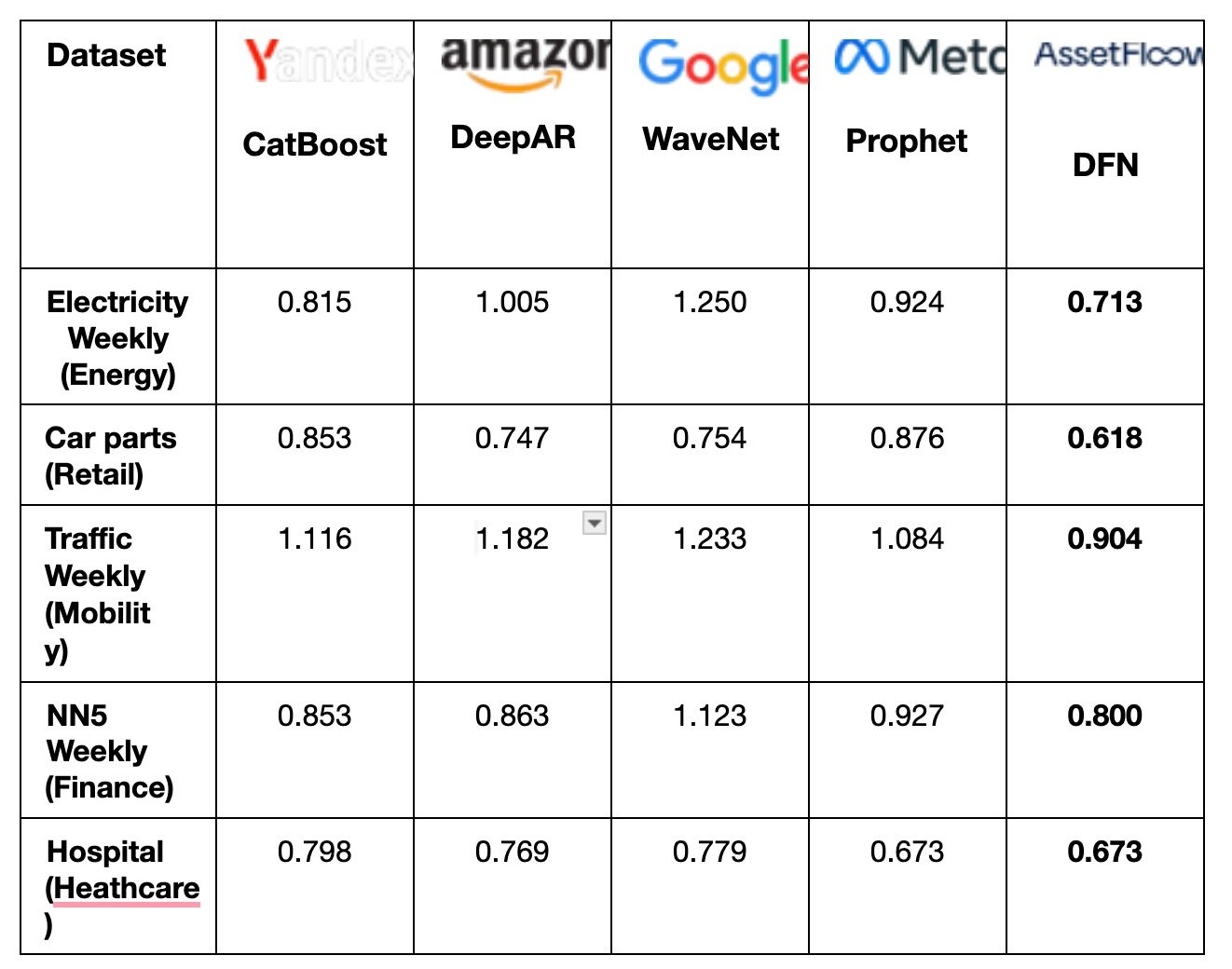
Ricardo Santos, the co-founder and CTO of AssetFloow, led the creation of the DFN which does not require feature engineering and learns the cause-effect of the data, over time.
Commenting on this achievement, Santos shared:
"Until today, no forecast model could outperform all dataset categories at once. Now, in its second generation, DFN has become the leader across all categories, showcasing its versatility to be trained with any type of dataset. This model is a game-changer."
In 2022, Ricardo conducted a test run of the first-generation DFN model on stock portfolio optimisation. The model exhibited an ability to identify optimal stock choices from NASDAQ and suggest when to buy"/sell.
According to eToro, the model's strategy yielded a lower Risk Score than the world's top three traders, achieving 79 per cent of profitable weeks when others did 40-45 per cent.
AssetFloow secured a €1.5 million investment in March 2023 to enhance its AI models applied to the retail sector, addressing the $1.5 trillion inventory issue known as the Ghost Economy.
Inadequate forecast models used by retailers primarily cause this problem. It contributes significantly to food waste. Leading retail giants, including EDEKA, Schwarz Group, REWE Group, ALDI, and Norma, have committed to reducing food waste by 30 per cent by 2025 and aim for an even more ambitious target of halving it by 2030.
Ricardo Santos explained:
"We started with retail because the current approach of selling excess food nearing expiration dates at lower prices is merely a band-aid solution.
The real solution lies in accurate and explainable forecast models to eliminate out-of-stocks and overstocks."
AssetFloow announced this year the launch of the DFN solution as APIs, to empower other companies to harness the forecasting capabilities of DFNs in entirely different markets beyond retail".
Lead image: Ricardo Santos, co-founder and CTO of AssetFloow. Photo: uncredited.
Would you like to write the first comment?
Login to post comments