Alexandra Ebert has possibly the coolest job title ever: Chief AI and Data Democratization Officer at MOSTLY AI.
With a Master's thesis in Machine Learning and the GDPR, Ebert brings deep expertise to her role, also chairing The IEEE Synthetic Data IC Expert Group (IEEE Standards Association) and hosting the Data Democratisation Podcast.
Following her master's, she began working at MOSTLY (founded in 2017), a synthetic data company that allows organisations to create fully anonymous datasets that retain the statistical properties of original data.
Its privacy-preserving synthetic data platform mimics real data without exposing sensitive information, with high-fidelity outputs that are recognised as some of the most accurate in the market, making them suitable for advanced AI and machine learning applications.
MOSTLY's platform enables organisations to safely unlock access to their sensitive data assets and realise the full potential of this data to drive AI innovations and, in doing so, address the problems with historical data anonymisation.
The company recently launched the first industry-grade open-source synthetic data toolkit (SDK), enabling any organisation to easily generate high-quality, privacy-safe synthetic datasets from sensitive proprietary data, all within their own compute infrastructure.
But before we dig into what it offers, let's explore why it's needed.
The problem with data anonymisation tech
According to Ebert:
"There are many anonymisation technologies out there, which shockingly are still used to date, even though researchers have been shouting for decades now that they're not privacy safe and GDPR compliant."
Traditional methods like masking and obfuscation belong to the era of small data. In the past, organisations only had access to a handful of data points per customer—perhaps basic demographic details and some account information. These techniques were inherently destructive when applied to the original dataset."
For example, a bank with a customer data table might redact sensitive details like last names and social security numbers using a black marker.
Even transaction details could be altered — your coffee at Starbucks might no longer be listed as $7 but instead as an estimated range of 5 to 10 euros or pounds.
"The goal was to obscure data until it seemed sufficiently anonymised.
However, research has repeatedly demonstrated that such methods are ineffective in the era of big data. Today, large enterprises typically hold hundreds, if not thousands or even tens of thousands, of data points per customer.
For instance, with credit card transactions, knowing just the merchant and the date of three separate transactions is often enough to re-identify 80 per cent of customers."
According to Ebert, the other problem is that "AI thrives on data. If an organisation originally had 10,000 data points per customer but was reduced to just three or five due to anonymisation, the overall value of the dataset would diminish significantly.
This creates a dilemma: businesses need high-quality data for insights and innovation, but traditional privacy protection methods compromise its usefulness."
The value of synthetic data
Unlike conventional techniques that modify, mask, or remove information from an existing dataset, MOSTLY's synthetic data platform leverages generative AI to analyse and understand the data's structure, patterns, and relationships.
"Put simply, an AI model can learn how customers of a particular bank, telecom provider, or health insurer behave over time—capturing trends, dependencies, and correlations.
For example, it can determine whether a customer who visits Starbucks in the morning will likely dine out for lunch or make a purchase on Amazon later in the day. These behavioural patterns can be automatically detected and replicated, preserving the statistical integrity of the data while ensuring privacy."
MOSTLY AI's tech incorporates a comprehensive set of privacy mechanisms to ensure that no personal secrets are learned or retained. The AI extracts generalisable patterns at a highly granular level while preventing the inclusion of uniquely identifiable individuals.
Ebert details:
"For instance, if the dataset included a highly distinctive individual—like Bill Gates — he would be excluded to prevent a privacy violation, especially in regions with fewer billionaires, such as Austria, compared to the United States.
Similarly, if there were only five individuals with an extremely rare disease, they would also be removed to safeguard their privacy.
However, when characteristics appear in larger groups—say 20, 30, or 50 individuals—those patterns can be retained while still ensuring privacy protection."
The process involves three key steps:
- Advanced AI-driven learning,
- Rigorous privacy mechanisms to filter out uniquely identifiable individuals,
- A completely separate generative process that creates synthetic data from scratch—without modifying or shuffling the original dataset.
This ensures both privacy protection and the preservation of valuable statistical insights.
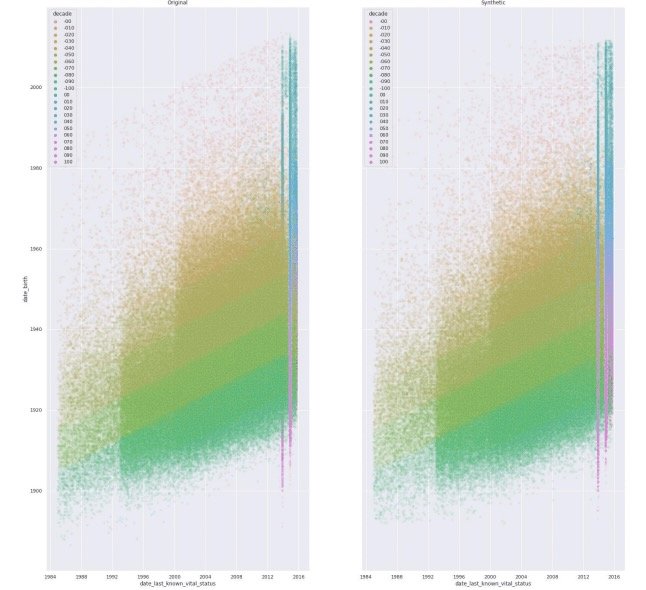
MOSTLY works with Fortune 100 businesses across Europe, North America, and Asia and has raised $31 million since its launch. Customers include CitiBank, the US Department of Homeland Security, Erste Group, Telefonica, and two of the five largest US banks.
A world-first open source toolkit for creating privacy-safe synthetic data.
As part of MOSTLY's tool kit, synthetic data SDK is available as a standalone Python package at https://github.com/mostly-ai/mostlyai under the fully permissive Apache v2 licence. What's more, it's easy to use.
Ebert shared:
"We ensure that our technology is super simple to operate because back in the day, with legacy anonymisation, you needed to be an expert. With Mostly AI, you don't need to decide how to protect privacy.
The mechanisms activate automatically for any given data set that you put in to ensure complete anonymity."
However, according to Ebert, while organisations strive for widespread data use in AI and innovation, data remains siloed and inaccessible to most employees, with gatekeepers lacking the motivation to share.
"In the past, data access was handled on a case-by-case basis. Enterprises would approach us with specific challenges—such as improving customer churn models that underperformed due to low-quality training data.
Strict regulations like GDPR prevented access to production data, so they sought synthetic datasets that were both privacy-compliant and high-quality."
Today, the shift is toward enterprise-wide data democratisation, enabling every employee to leverage AI effectively, with executives aiming to augment technical teams and marketing, sales, and other business units.
The value of open source
According to Ebert, open source plays a crucial role in MOSTLY's mission to democratise data:
"It was always our mission to democratise data, and we believe that this is such an important resource that we need to open up data access not only within businesses but also society at large."
Mostly works with "ginormous" Fortune 100 companies, and using open source tech makes it much easier for customers to deploy it in any environment, test it out, and then organically grow within an organisation.
Ebert asserts:
"We can talk about AI saving the world, curing cancer, and helping tackle the climate crisis all day. If you're not going to open up data to the general public, NGOs, and researchers, the aspiration will not become a reality.
If data is hoarded within the big corporates, the big techs, they always have for-profit motives, and we will not really use AI for societal progress.
For example, we also want to integrate more tightly with leading cloud providers and open source helps there."
How synthetic data can fuel startup innovation and enterprise collaboration
According to Ebert, being an AI ethicist at heart means ensuring responsible AI practices — transparency, fairness, and privacy — are built into inventions from the start, not treated as an afterthought.
She notes that many startups developing products for enterprises lack their own datasets, and "traditional methods can take months to produce incomplete and insecure "Swiss cheese" anonymised datasets."
"Traditional anonymisation methods take months and still result in incomplete, low-value datasets that may not be fully secure. Synthetic data reduces this process to just one or two business days, allowing companies to quickly and safely share data."
She advises startups should proactively request synthetic data:
"If a bank provides a synthetic version of its financial transactions, both parties benefit—the startup can build better products, and the bank gains more effective innovation.
They can develop better products, and enterprises interested in bringing in startup innovation always need data to validate it."
Would you like to write the first comment?
Login to post comments